Abstract
An important problem in reinforcement learning is designing agents that learn to solve tasks safely in an environment. A common solution is to define either a penalty in the reward function or a cost to be minimised when reaching unsafe states. However, designing reward or cost functions is non-trivial and can increase with the complexity of the problem. To address this, we investigate the concept of a Minmax penalty, the smallest penalty for unsafe states that leads to safe optimal policies, regardless of task rewards. We derive an upper and lower bound on this penalty by considering both environment diameter and controllability. Additionally, we propose a simple algorithm for agents to estimate this penalty while learning task policies. Our experiments demonstrate the effectiveness of this approach in enabling agents to learn safe policies in high-dimensional continuous control environments.
Publication
Reinforcement Learning Safety Workshop at RLC
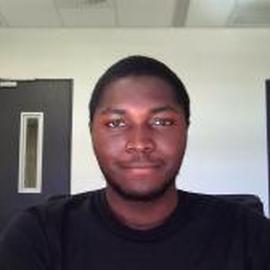
Associate Lecturer
I am an IBM PhD fellow interested in reinforcement learning (RL) since it is the subfield of machine learning with the most potential for achieving AGI.
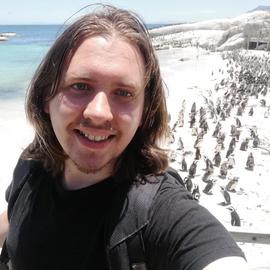
PhD Student
I am a PhD student at the Institut de Robotica i Informàtica Industrial (IRI) (under CSIC and UPC) in Barcelona, working on the TRAIL Marie Skłodowska-Curie Doctoral Network under the supervision of Guillem Alenyà. I was previously an MSc student and lecturer at the University of the Witwatersrand, under the supervision of Benjamin Rosman and Ritesh Ajoodha, as well as a member of the RAIL Lab.
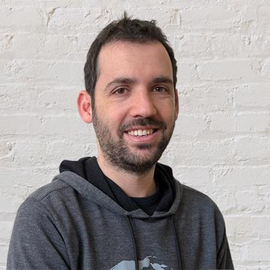
Deputy Lab Director
My research interests include reinforcement learning and planning.
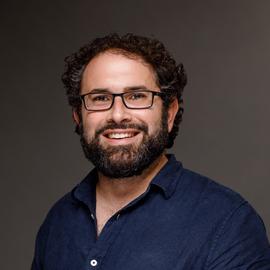
Lab Director
I am a Professor in the School of Computer Science and Applied Mathematics at the University of the Witwatersrand in Johannesburg. I work in robotics, artificial intelligence, decision theory and machine learning.