Abstract
We propose a framework for defining a Boolean algebra over the space of tasks. This allows us to formulate new tasks in terms of the negation, disjunction and conjunction of a set of base tasks. We then show that by learning goal-oriented value functions and restricting the transition dynamics of the tasks, an agent can solve these new tasks with no further learning. We prove that by composing these value functions in specific ways, we immediately recover the optimal policies for all tasks expressible under the Boolean algebra.
Publication
Beyond Tabula Rasa in Reinforcement Learning (Workshop at ICLR)
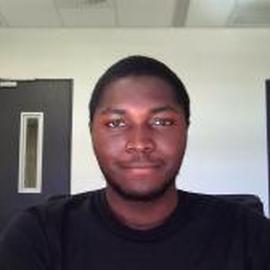
Associate Lecturer
I am an IBM PhD fellow interested in reinforcement learning (RL) since it is the subfield of machine learning with the most potential for achieving AGI.
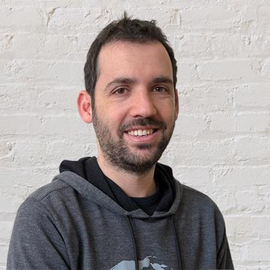
Deputy Lab Director
My research interests include reinforcement learning and planning.
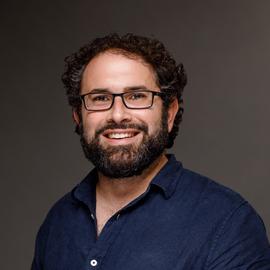
Lab Director
I am a Professor in the School of Computer Science and Applied Mathematics at the University of the Witwatersrand in Johannesburg. I work in robotics, artificial intelligence, decision theory and machine learning.